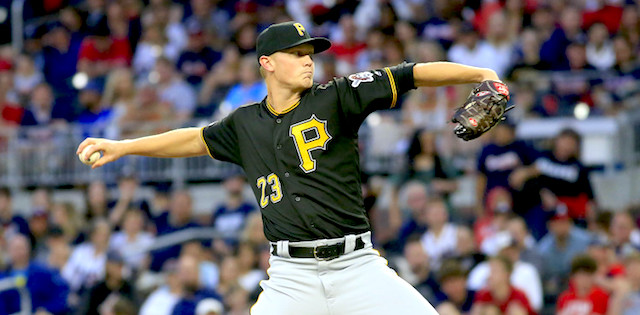
Spring is in the air and MLB regular season is only a few weeks away! Hopefully, you're using our RotoBaller rankings and preseason content to crush your season-long drafts and build some winners in your season-long leagues.
If you haven't already read it, check out Jon Anderson's article about stacking hitters for GPPs and some great work of his here about DFS pitchers - and keep watching as we are going to be releasing more MLB DFS strategy articles leading up to opening day on April 1st! If you ever want to chat with me about MLB, DFS, or fantasy sports in general, hit me up on Twitter @ThunderDanDFS.
In this series of articles, I am going to try to lay out a number of overlooked factors that can be exploited by savvy MLB DFS players. Baseball is such a stat-nerd sport, and there are so many different statistics that one can consider when trying to break down any slate of games. I'll cover the basics for both hitters and pitchers in my annual DFS Strategy guide, but for today I want to focus on an often under-analyzed angle - and that's how to identify pitchers who are bound for regression (so that we can target them in DFS).
Featured Promo: Get any DFS Premium Bundle for for 30% off using code NEW! Win more with expert advice from proven winners and exclusive DFS tools. Get instant access to RotoBaller's Lineup Optimizers, Research Stations, daily picks and VIP chat rooms across 10 sports! Go Premium, Win More!
Stats to Use When Looking For Regression Candidates
Stacking against a pitcher who is coming into their start with a 6.00 ERA and has allowed 10 homers in 8 starts is easy. It's almost too easy, meaning the opposing offense is going to be an obvious stack for tournaments (and perhaps still the right one). But baseball is a game filled with variance and the likelihood of that pitcher with terrible stats actually being decent on any given day exists. One of the best ways to differentiate your lineups is to find pitchers whose surface numbers don't look bad, but whose advanced statistics scream that negative regression is coming. Here are some of the stats that I look at when trying to identify those pitchers on a daily basis.
- ERA estimators - SIERA and xFIP: These are both better indicators of a pitcher's performance than ERA, which is an inherently flawed statistic. SIERA stands for "Skill-Independent Earned Run Average" and XFIP stands for "Expected Fielding Independent Pitching." Both metrics give a more comprehensive look into how a pitcher performed than just how many runs they allowed and factor in variables that ERA doesn't (such as luck, defense, park factors, etc...)
- BABIP (Batting Average on Balls in Play): This stat can be used for hitters and pitchers, but can help in determining how lucky or unlucky a pitcher has been with how often they get outs on their pitches that are put into play by hitters.
- WHIP (Walks + Hits Allowed per Inning Pitched): This lets us know how many baserunners a pitcher is allowing on a per-inning basis.
- LOB% (Left On Base Percentage): This is often known as a "strand rate" and refers to how often pitchers strand runners on base to end an inning (they have to be runners that they allowed on base, not inherited runners).
- HR/FB rate (Homerun to Fly Ball ratio): This simply tells us how often a pitcher gives a home run on a fly ball (not all home runs are captured by this statistic as some are line drives).
What I did was go through the 2020 data for all starting pitchers who threw at least 20 innings and took a look at each of these statistics to see who the outliers were. Since we had a small sample size of data in 2020 due to the short season, the data set is almost perfect for what I am trying to show here. Imagine that we were 50 games into this season and had this data from the first games to use in order to draw some conclusions about who was overperforming based on their surface numbers - and those are the pitchers we would want to pick on.
SIERA and xFIP
Wow, I knew Mitch Keller wasn't as good as his numbers suggested, but I wasn't prepared for often I was going to see him on these lists. Keller stands out here with a four-run difference in his ERA and SIERA and would be a prime candidate to pick on. I am a Pirates fan and I want to think he's on the verge of breaking out, but the data suggests he was hit hard and got incredibly lucky that he didn't have another ugly ERA in 2020, which was the opposite of 2019 when he had terrible surface numbers despite some encouraging signs.
It's a lot of the same names here when we pull the largest differences in xFIP and ERA with Keller and Kim again leading the way. Urquidy was another fraud who I was stacking against and Brault is a guy I have never been a believer in (perhaps I am just a cynical Pirates fan). A few bigger names show up on this list with Bauer overperforming his xFIP and Braves' youngster Ian Anderson, though neither of them are really guys to pick on in DFS (but I did see some of our MLB guys talking about Bauer as being overrated in season-long fantasy this season).
BABIP
Guess who shows up again when we look at the lowest BABIP numbers on the season? Yep, Mitch Keller. He was extremely lucky on batted balls. For reference, the average BABIP is usually around .300 for pitchers and the average of all the data I used when compiling these charts was .286. It's not a surprise to see Dunn and Javier here either as both of them had big gaps in ERA vs. xFIP like Keller. Hudson is a guy you can usually target with lefties, but who is solid against righties and gets ground balls. Buehler is good but wasn't as dominant as we had seen him be in the past.
LOB%
Mitch Keller is our winner, again! With a strand rate near 94%, no wonder he kept that ERA low. As you look down this list, there are a lot of good pitchers here and strikeout pitchers. Having a high strand rate isn't necessarily a bad thing, because good pitchers find ways to get out of innings. But when you add the SIERA into that chart you can see which of these guys were legit (Cole, Bauer, Bieber) and which of them were just getting lucky to get out of innings unscathed or with minimal damage. Use this statistic with caution because it obviously doesn't tell the whole story, but paired with some of this other data it can help us see which pitchers are getting out of dangerous situations more often than they should be. For reference, the average strand rate from this data set was 72.8%
HR/FB%
I want dingers from my stack, and I want to find pitchers who are going to give up multiple bombs to my hitters. Looking at HR/FB rates allows us to see which pitchers are giving up more (or less) than they really should be. The average rate for this data set was 15.7% and I pulled only those pitchers who were under 9% last season to see what we could find.
I included each pitcher's xFIP and FB% to give us a clearer picture. If a guy isn't a fly-ball pitcher, then I am probably not targeting him that often in the hopes of getting multiple homers anyway. So that takes guys like Zach Wheeler (elite groundball guy), Max Fried, Dallas Keuchal, Ian Anderson, Brad Keller, and Pablo Lopez out of the picture as they have low FB rates. Look at Michael Pineda though! He's a fly ball pitcher who didn't give up a single homer in 26 innings! I would absolutely be stacking against him the next time he pitched. The same goes for Tyler Anderson, Corbin Burnes, Dylan Bundy, and Julio Urias - who all fit the mold here of guys who should have given up more homers based on the frequency of fly balls allowed vs. the lack of homers allowed.
Well, I hope this information helps you make more informed decisions this season and make sure that you continue to follow our MLB DFS strategy articles throughout the season!
Download Our Free News & Alerts Mobile App
Like what you see? Download our updated fantasy baseball app for iPhone and Android with 24x7 player news, injury alerts, sleepers, prospects & more. All free!
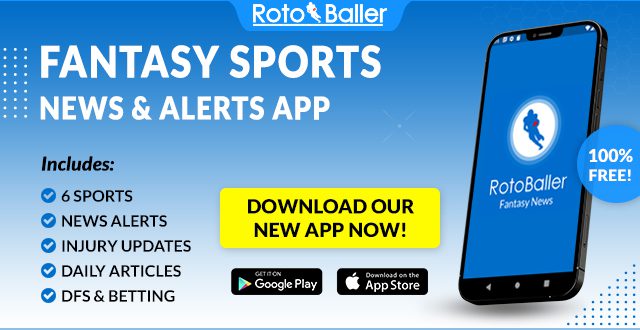